A Detailed Overview of AI and ML Solutions for Healthcare
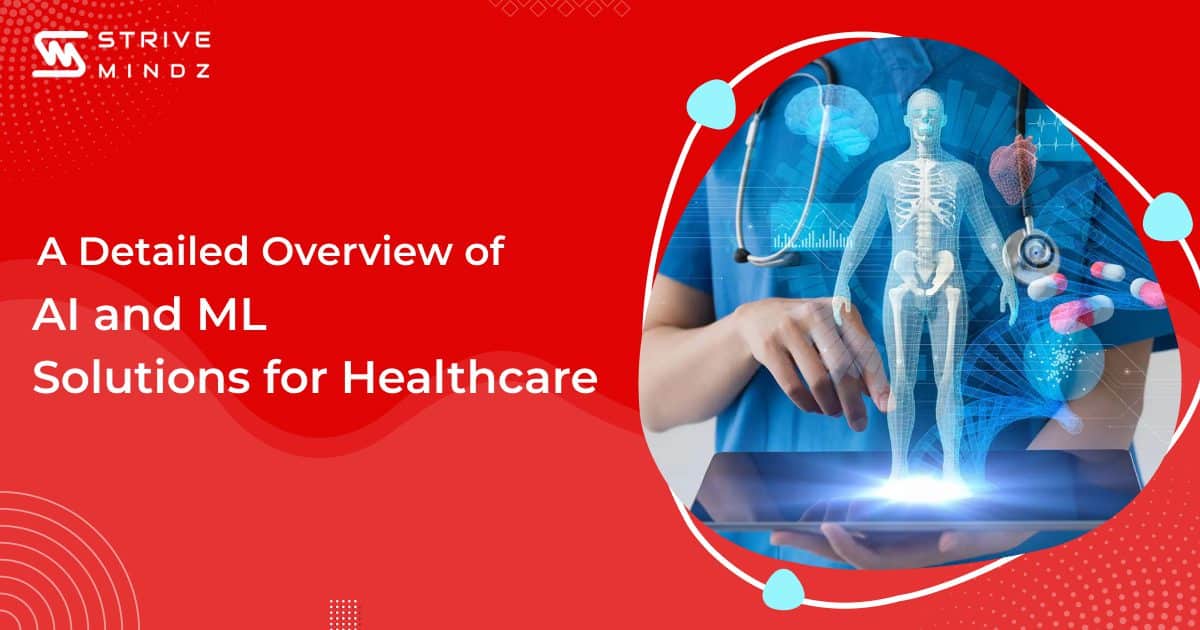
Artificial Intelligence (AI) and Machine Learning (ML) are transforming the healthcare industry, ushering in a new era of improved patient care quality and efficiency. The healthcare industry produces an enormous volume of data and with the advent of these technologies, healthcare professionals now have access to the tools they need to diagnose complex illnesses, develop customized treatment plans and optimize healthcare operations.
This comprehensive guide delves into the breadth of AI and ML in healthcare, exploring the latest advancements and innovations, ethical considerations and limitations as well as real-world success stories of these technologies in action. By leveraging AI and ML healthcare providers can enhance patient outcomes, reduce costs and improve overall healthcare delivery. From predictive analytics to image recognition, AI and ML are revolutionizing the way healthcare professionals approach patient care.
However, with any emerging technology, some ethical considerations and limitations must be taken into account. This guide provides a thorough examination of these issues ensuring that healthcare professionals are equipped to navigate the complex landscape of AI and ML in healthcare.
Defining AI and ML in Healthcare
These technologies are transforming the way doctors diagnose and treat patients, cutting costs and improving patient outcomes. These can be used in various healthcare applications, including medical imaging, clinical decision support, drug discovery and personalized medicine. The application of these technologies in healthcare is expected to grow exponentially in the coming years.
In healthcare, AI can be used to analyze complex medical data, predict outcomes and assist doctors in making more accurate diagnoses. On the other hand, ML refers to the ability of machines to learn from data and improve their performance without being explicitly programmed.
In healthcare, ML can be used to identify patterns in medical data, evaluate the effectiveness of treatments, and personalized medical care for each patient. Together, AI and ML can help to make healthcare more efficient, effective, and personalized.
Artificial Intelligence (AI) & Machine Learning (ML) Market Size, Growth, Report 2023 – 2030
-
Artificial intelligence (AI)
A total of USD 428.00 billion will be available in the artificial intelligence market in 2022. The market is expected to grow from USD 515.31 billion in 2023 to USD 2,025.12 billion by 2030, exhibiting a compound annual growth rate of 21.6%.
Through the creation of intelligent software and hardware, artificial intelligence simulates human intelligence processes by replicating human behaviour like learning and problem-solving using a variety of machines. There are several Al-based solutions covered in the report, including AWS Chatbots, OpenAl Codex, and Azure Al, among others.
Due to the increase in artificial intelligence applications, the growth of relevant partnerships and collaborations, the rise of small-scale Al providers, the changing complexity of business structures, and the demand for hyper-personalized services, the global market is set to grow dramatically. As well as government initiatives and investments in Al technologies, end users and enterprises benefit from them.
-
Machine Learning (ML)
According to market estimates, the global market for Machine Learning (ML) will reach USD 19.20 billion by 2022. During the forecast period, the market is expected to grow from USD 26.03 billion in 2023 to USD 225.91 billion by 2030, exhibiting a CAGR of 36.2%.
The field of machine learning comes under the umbrella of Artificial Intelligence (Al). The method teaches computers to learn from algorithms and data and quickly mimic the way humans learn. The market will grow as Al and ML technologies are adopted by a wide range of end-use industries, including healthcare, automotive, retail, and manufacturing. Additionally, several countries adopted quarantine measures and social distancing policies to mitigate the impact of COVID-19 in 2020. In order to analyze the effects of these policies and measures, developers and researchers planned to use machine learning tools.
Moreover, the global geriatric population is increasing and changing lifestyles have led to a rise in chronic diseases. As a result, there is a growing demand for early diagnosis and improved understanding of diseases in their initial stages. To meet this demand, healthcare systems are increasingly adopting algorithms of these technologies to accurately predict diseases based on historical health datasets.
Advancements in Medical Diagnosis and Treatment with AI
-
Diagnosing Diseases with AI
One of the most significant benefits of AI in healthcare is its ability to assist in diagnosing diseases. AI tools can analyze vast amounts of medical data, providing doctors with insights and predictions about a patient’s condition. For example, AI can help to identify early signs of diseases such as cancer, heart disease and Alzheimer’s.
-
Improving Precision Medicine with Machine Learning
Medical treatments are adjusted to meet the specific requirements of each patient with precision medicine. It involves analyzing a patient’s genes, environment and lifestyle to develop personalized treatments. ML can help to identify patterns in this data and predict which treatments will be most effective for each patient.
For example, ML algorithms can analyze a patient’s genetic information to identify the mutations that are driving their cancer. This information can then be used to develop a personalized treatment plan that targets those mutations.
Cutting Costs and Improving Patient Outcomes with ML in Healthcare
-
Reducing Medical Errors with AI
Medical errors are a significant problem in healthcare. They can result in patient harm, increased costs and decreased quality of care. AI can help to reduce medical errors by providing doctors with real-time information about a patient’s condition, alerting them to potential problems and providing decision support.
For example, AI can be used to review a patient’s medical history and identify potential drug interactions or allergies. This information can then be used to guide treatment decisions and reduce the risk of adverse events.
-
Increasing Efficiency and Productivity in Healthcare
Another significant benefit of ML in healthcare is its ability to improve efficiency and productivity. ML algorithms can automate repetitive tasks, such as data entry and free up doctors and nurses to focus on patient care. Additionally, ML can help to optimize hospital operations, such as scheduling, staffing, and inventory management.
Ethical Considerations and Limitations of AI in Healthcare
-
Ensuring Data Privacy and Security in Healthcare
One of the most significant ethical considerations in the use of AI and ML in healthcare is data privacy and security. Safeguarding sensitive patient data from illegal access or usage is essential for protecting their welfare and privacy. Healthcare organizations must implement robust data security protocols to ensure patient’s information safety.
-
Addressing Bias and Fairness Issues in AI and Machine Learning
Bias is a significant concern in the use of AI and ML in healthcare. Biased models can lead to inaccurate diagnoses or treatment decisions, particularly for underrepresented groups. Healthcare organizations must take steps to identify and mitigate bias in their AI and ML models.
Additionally, AI and ML technologies are not a replacement for human decision-making. Doctors and other healthcare professionals should maintain their judgment and expertise in healthcare decision-making, even when assisted by AI and ML tools.
Emerging Trends and Future Directions of AI and ML in Healthcare
As AI and ML continue to make strides in healthcare, emerging trends and future directions show great potential for improving patient outcomes. One trend is the use of natural language processing to analyze medical records and data, making it easier for physicians to identify patterns and diagnose illnesses. Another trend is the development of virtual assistants and chatbots to help patients with scheduling appointments and answering basic medical questions.
However, navigating regulatory changes and standards remains a challenge for AI and machine learning developers in healthcare. As these technologies continue to evolve, regulatory bodies must keep up with the pace of innovation to ensure patient safety and accuracy in diagnoses.
Exploring opportunities for AI and machine learning in unconventional healthcare areas, such as mental health, rehabilitation and assistive technologies, also holds great potential for fostering positive health outcomes.
-
Integration of AI and ML in Healthcare
Developing a strategy for adopting AI and machine learning in healthcare requires careful consideration of factors such as data privacy, security and ethics. It is also crucial to involve stakeholders from various departments and specialities to ensure the technology is implemented effectively.
Despite the benefits of AI and machine learning in healthcare, challenges in implementation exist. These challenges include a lack of standardized data and difficulty in integrating the technology with existing healthcare systems. Overcoming these challenges requires collaboration, investment in infrastructure, and a willingness to adapt to change.
-
Innovations in Medical Research with AI
AI and machine learning have the potential to revolutionize medical research by speeding up drug discovery and development, as well as improving clinical trial design and execution. By analyzing vast amounts of data, algorithms of these technologies can identify patterns and relationships that may not be visible to humans, leading to insights that could dramatically alter the course of medical research.
AI can also be used to identify new drug targets and develop personalized treatment plans for patients with a variety of conditions. Improving clinical trials with machine learning allows researchers to identify which patients are most likely to respond to a particular treatment, leading to more efficient and effective research.
Success Stories of Successful Application of AI in Healthcare
Real-world success stories of implementing AI and machine learning in healthcare demonstrate the enormous potential of these technologies. Examples include predicting which patients are at risk for hospital readmission, reducing diagnostic errors and improving the accuracy of breast cancer screening.
AI and ML have numerous use cases in the healthcare industry. One of the most significant applications of AI and ML in healthcare is in the diagnosis of diseases. AI algorithms can analyze large amounts of data from medical records, lab tests, and imaging scans to identify patterns and make accurate diagnoses. For example, Google’s DeepMind Health uses machine learning algorithms to analyze retinal scans and diagnose diabetic retinopathy.
The use of AI and ML is expanding in healthcare, particularly in customizing treatment programs for patients. AI algorithms can analyze patient data, including genetics, medical history and lifestyle factors to identify the most effective treatments for each patient. The potential for enhanced outcomes and minimized adverse reactions is present.
Conclusion
To sum up, AI and ML have tremendous possibilities in healthcare but, facing the barriers that arise with their application is vital. Healthcare providers must prioritize ethical considerations and patient data privacy and security.
Additionally, consumers will play a crucial role in shaping the future of healthcare technology, and healthcare providers must be prepared to meet their demands. Collaboratively, we can utilize AI and ML to enhance healthcare results and revolutionize the field.
Strivemindz is making significant strides in leveraging AI, ML, and IoT to become a game-changer in various industries, including healthcare. Our team is dedicated to ensuring that these cutting-edge technologies are effectively integrated into businesses to enhance their operations and improve outcomes.
The amalgamation of our deep-rooted knowledge and unceasing pursuit of innovation has bolstered our confidence in transforming customary business practices and bestowing value upon their beneficiaries.
Frequently Asked Questions
[faq-schema id=”3795″]